Poster: Time-Efficient Sparse and Lightweight Adaptation for Real-Time Mobile Applications
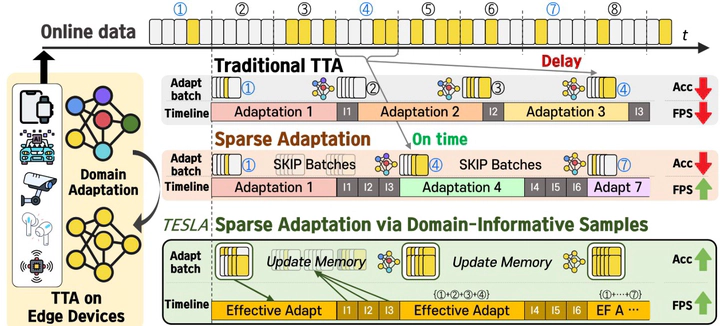
Abstract
When deployed in mobile scenarios, deep learning models often suffer from performance degradation due to domain shifts. Test-Time Adaptation (TTA) offers a viable solution, but current approaches face latency issues on resource-constrained mobile devices. We propose TESLA Time-Efficient Sparse and Lightweight Adaptation strategy for real-time mobile applications, which skips adaptation for specific batches to increase the inference sample rate. Our method balances model accuracy and inference speed by accumulating domain-informative samples from non-adapted batches and sparsely adapting them. Experiments on edge devices demonstrate competitive accuracy even with sparse adaptation rates, highlighting the effectiveness of our approach in real-time mobile applications. Our strategy can seamlessly integrate with existing lightweight adaptation and optimization algorithms, further accelerating inference across diverse mobile systems.
Type
Publication
The 22nd ACM International Conference on Mobile Systems, Applications, and Services (MobiSys ‘24)